Chris Sheehan, SVP Strategic Accounts and AI, Applause
The financial services industry sees great promise in generative AI (Gen AI). It’s viewed as a transformational force capable of driving significant value through innovation and improved efficiency. However, its adoption is not without challenges.
The technology is part of a broader spectrum of AI technologies, sitting alongside traditional AI and advanced AI. While Gen AI offers significant potential benefits, traditional AI, such as predictive machine learning models, encounters fewer hurdles and has the potential to drive even higher value.

McKinsey’s recent forecast underscores this potential, estimating that banks could unlock between $200 to $340 billion in value through the adoption of Gen AI. Traditional AI and analytics are expected to drive hundreds of billions of dollars in cost savings and revenue lift greater than Gen AI. While Gen AI technologies are new and more opportunities may be identified over time, traditional AI solutions already have a lot of value to offer and have still not reached full adoption inside financial services organizations.
Unpacking the Benefits and Challenges of Generative AI
Benefits
- Enhanced Customer Experience and Support Savings: Gen AI enables personalized banking services, significantly improving customer self-service success rates, reducing call times, and streamlining customer service operations.
- Fraud Detection and Risk Management: By analyzing patterns in data, Gen AI offers a robust solution for identifying potential fraudulent activities that leave evidence in text-based data, thereby enhancing security and reducing fraud-related losses.
- Streamlined Operations: The adoption of Gen AI enhances service and operational efficiency by processing and analyzing large volumes of unstructured data with speeds unmatched by human analysts.
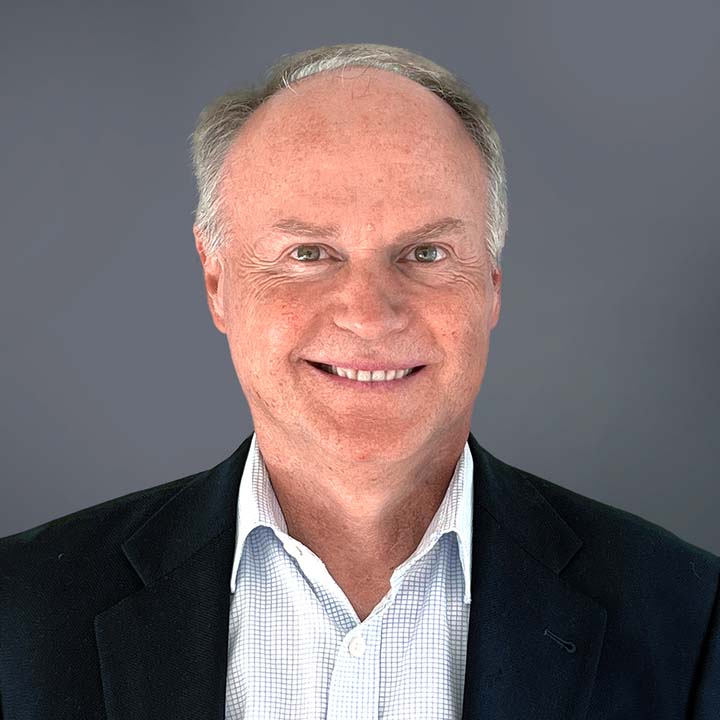
Challenges
- Data Privacy and Security: The integrity and confidentiality of customer data are paramount, requiring well-tested guardrails that prevent specific confidential information from being shared publicly. In addition, Gen AI solutions expose a new type of security vulnerability with open-ended prompt boxes, models that expect to receive a wide variety of instructions and variable responses.
- Regulatory Compliance: There is no clear playbook for Gen AI compliance. The dynamic regulatory landscape will require AI system updates and ongoing testing as evolving legal standards and regulations are put in place.
- Bias and Fairness: Ensuring AI algorithms are free from biases is crucial to prevent unfair treatment of customers, highlighting the importance of extended risk testing for AI safety and ethics.
- Integration and Implementation: Integrating AI into existing systems is complex and can pose challenges. A lack of data readiness for Gen AI is common and requires attention before teams can push solutions to production.
These challenges listed above are surmountable. However, teams must consider their mitigation strategies and testing protocols to address these potential issues as part of Gen AI planning.
Beyond Generative AI
While Gen AI is capturing the limelight for its potential to revolutionize the financial services sector, teams must not overlook the complementary roles played by traditional AI and predictive machine learning (ML) models. These offer distinct advantages and pose fewer challenges than Gen AI.
Predictive ML models are adept at finding signals in noise and provide invaluable insights for decision-making processes, while traditional AI (which includes conversational AI) enhances customer interaction with more responsive and intuitive services. These technologies are not in competition, but rather complementary components of a comprehensive AI capabilities strategy.
Successful implementation in financial services typically includes a blend of Gen AI with chatbots that use natural language processing (NLP) for customer engagement and ML predictions for analytical depth. This multifaceted approach enables financial institutions to leverage the unique strengths of each AI technology, ensuring a robust, flexible and efficient digital infrastructure capable of adapting to future challenges and opportunities.
Refining Your AI Capabilities
In navigating the complex landscape of AI technology, choosing the right tools and models is just the beginning. The effectiveness of these technologies hinges on the quality of data collection, training and testing, for which a concept known as red teaming plays a pivotal role.
In red teaming, experts attempt to exploit vulnerabilities in a system, simulating potential threats to identify weaknesses. This method originated in the cybersecurity industry and addresses safety, privacy and accuracy. The ultimate goal is to ensure AI systems not only perform their intended functions, but do so in a manner that is secure, fair and aligned with ethical standards.
Gen AI is a top agenda item for many organizations. When adopting the technology, it is important to address key challenges, and testing with real users is an important element. At the same time, also don’t forget about traditional AI and machine learning solutions. It is likely that your organization has only scratched the surface of the benefits that less flashy versions of AI technology can deliver.