By Brian Unruh is Chief Financial Officer (CFO) at ABBYY
When you hear your dentist talk about the use of artificial intelligence, you know it’s permeated every aspect of our day-to-day life. Yet, you’ve probably already had more intense c-suite discussions about the product roadmap, resources, and the ever-important topic – budget. It’s imperative for the CFO office to understand how AI can impact budgets – and not just in spending.
Relating to spending, a recent global survey by ABBYY reported that 82% of enterprise leaders increased their AI budgets in 2023. Most attributed a 2x return on investment and notable impact to accelerating product quality, delivering value to customers, and improving workers’ productivity. IDC affirms this trend and forecasts AI software revenue will grow to $251 billion by 2027. It sounds like a no-brainer to jump on the AI bandwagon, but not all AI is created equal. To contribute meaningful discussion to AI budget considerations, here are a few important factors CFOs should know before they approve AI spending.
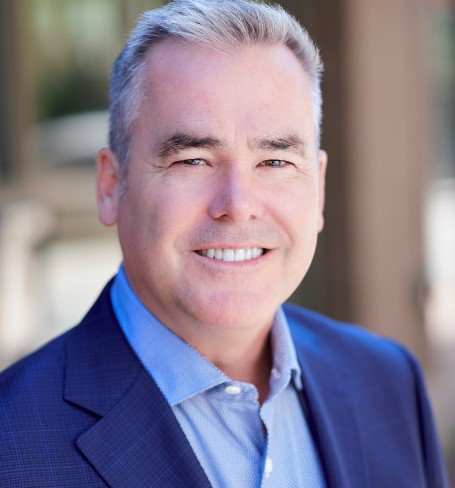
Insist on purpose-built AI
Generic AI tools like ChatGPT can be alluring. There is so much anticipation for them in the market that IDC estimates generative AI spending will reach $143 billion in 2027. However, tools like ChatGPT are constructed of large language models (LLMs) built on one trillion-plus parameters (equivalent to over 1 million books) and optimised for everything but what would help the finance office the most – a document.
As a result, generic AI tools can provide slower performance in processing the output you want, waste energy, and significantly waste power consumption to the equivalent of powering a small island. Suppose all these side effects weren’t already unacceptable within organisations trying to minimise costs and risks. In that case, it can deliver inconsistent results and even hallucinations, making it difficult for humans in the loop to know when an answer is correct and how to address the error so it does not repeat.
Alternatively, purpose-built AI narrows the context and task to the core of what it needs, so it achieves the results the business needs. That also has been tested against a focused data set of the world’s most complex documents rampant throughout enterprises. These include invoices, POs, customer correspondences, claims forms, tax forms, and hundreds of other documents that drive a business.
When talking with your innovation leaders and technology partners, ensure the AI they recommend is purpose-built for the specific business problem and has proven results that deliver on a consistent basis.
Approve AI for revenue-generating processes
Economic concerns with inflation and high-interest rates compounded by a reduced labor force do not leave room for technological trial and error. Unless your organisation is developing and testing AI models, your IT budget should incorporate tech investments leveraging AI to improve the customer experience, operational excellence, and revenue.
Prudent CFOs have the fiduciary responsibility to advocate for AI tools to augment workers’ productivity and accelerate the straight-through processing of any business process impacting revenue. Ernst & Young states their clients leverage AI to streamline their operations at the front or back end.
For example, the heart of every organisation is accounts payables. Using purpose-built AI to streamline the processing and timing of invoice payments and purchase orders will greatly improve cash cycle outcomes. In this scenario, AI-powered document processing will read, understand, and classify critical financial data into ERP systems.
In other industries, such as healthcare, AI can expedite the review of medical records to approve patient referrals to see specialists and receive life-saving medications. For manufacturers and fast-moving consumer goods (FMCG) producers, customs clearance can be expedited across borders to get products to store shelves and consumers’ tables faster.
Understand the actual cost of AI
Whenever a new tech trend emerges, you risk vendors overpromising capabilities. Ensure the details are noted in service level agreements about performance, compute utilisation and precision of outcomes. Also, consider the cost for self-training generative AI models varies from as low as MosaicML’s model at $325K to $6.75M for Google’s PaLM model and as much as $100K a day for OpenAI’s ChatGPT. While the usage costs for ChatGPT Plus are available by a subscription-based model, consider the business use case and the number of users needed to complete enterprise-level document processing.
As mentioned, purpose-built AI can put enterprises’ information to work at scale at significantly lower costs and higher-level valued outcomes and consistencies.
The democratisation of AI means solutions should be available in low-code/no-code applications that can plug into your enterprise systems with pre-built model training available out-of-the-box. For example, AI used in document processing can identify, understand, and classify data within invoices with up to 95% accuracy and automatically process them 81% faster than humans. Furthermore, it has been proven to increase employee productivity by up to 400% and lower processing costs by 91%. These cost savings can be directly tied to project ROI and profitability.
Overall, CFOs can be more confident in approving AI budgets by getting answers to the following questions:
- Does the AI solution address the business problem or opportunity?
- Can the AI vendor provide evidence of success?
- Is the AI solution rooted in ethical and responsible practices?
- Does the vendor offer ongoing support and improvement post-adoption?