Alok Rustagi, Vice President, Data & Analytics, EXL
We’ve all seen the recent hype surrounding ChatGPT and the potential artificial intelligence has to transform our daily lives. But increasingly, businesses (and financial services in particular) are debating how similar algorithms could be used in more professional settings.
Machine learning and AI technology have the potential to be huge differentiators for banks – both operationally, and the impact on the bottom line – but they need large amounts of data if they’re to be effective. With interactions moving increasingly online, paired with large-scale modernisation efforts, banks are getting access to a wealth of data on their customers, but with legacy technology still holding many bigger banks back, fast-growing fintechs are leveraging this opportunity better. To bridge this gap, high street banks are investing millions to build machine learning and artificial intelligence (AI) capabilities, and to modernise their data assets and platforms. However, there are many considerations before AI and machine learning can become mainstream for retail lending decisioning – especially given the attention badly executed AI programs that are creating bias have received recently.
Using AI to help customers whilst remaining compliant
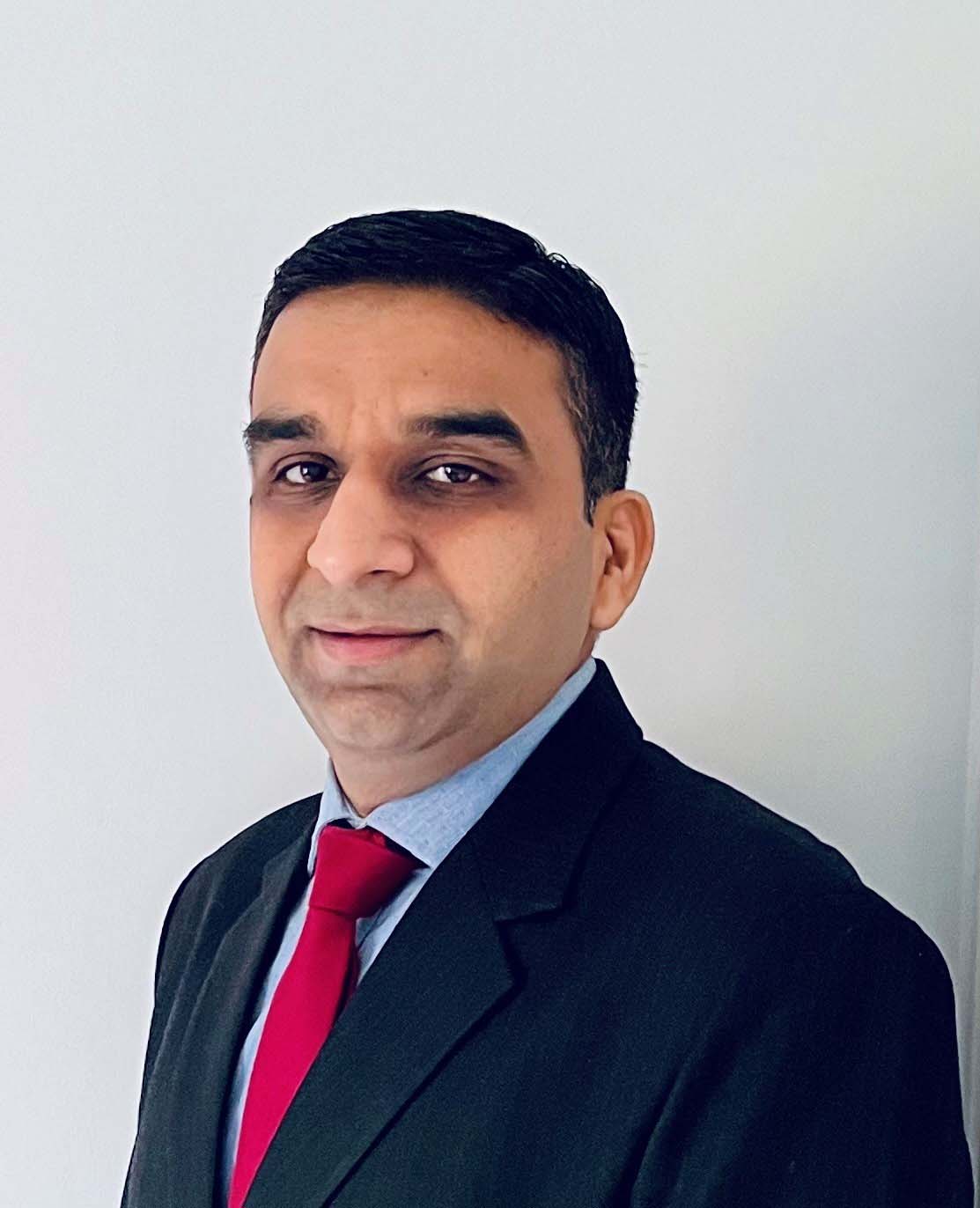
Unlocking value from data requires sophisticated analytics capabilities. Only proper tools and algorithms can trawl through a sea of data to pull only the actionable insights.
Building these capabilities from scratch is a huge challenge for many financial institutions already struggling with legacy technology or burdensome compliance requirements. A more manageable way to coordinate a digital transition is to look to technologies that can be plugged into a bank’s existing framework, at least in a transition phase.
AI-based solutions can be implemented to collate and interpret a bank’s customer data to present back a comprehensive view of its customers, on an individual basis. Rich insights can be drawn from payments data and bank account transactions to provide a customer’s complete financial profile. Detailed customer profiles can arm businesses with the insights they need to not only create tailored products for their customers, but also means they stay on the right side of regulation that’s increasingly focused on customer outcomes – the FCA’s Customer Duty legislation for example.
This also allows banks to support their customers in the best possible manner. In the face of a cost-of-living crisis, new predictive machine learning models will allow banks to prevent consumers running into major financial trouble. For example, by anticipating financial distress and intervening prior to a customer defaulting on a payment or falling into debt.
Using machine learning responsibly
In partnership with developments in AI, machine learning has embedded itself across the banking industry. From automating back-office processes, to improving accuracy, to managing and reducing fraud, it has already proved its potential to transform operations.
This ultimately puts banks and other financial institutions in a much stronger position to add value across the customer lifecycle by making sense of consumer needs, product usage, and credit profile. From risk underwriting controls and models to improving marketing effectiveness, AI solutions can transform a business’s efficiency.
But some thorny questions remain about how best to adopt machine learning for more complex processes and journeys – for example, approving applications, gauging risk, or making credit limit decisions. There’s a risk that without proper frameworks and governance structures, issues of inherent bias may creep in, and a bank’s ability to account for uncertainty or explain the rationale behind AI decisions might be compromised.
To balance this risk, data input must be qualified to reduce that risk, and machine learning must go hand in hand with cutting edge analytics and intelligence capabilities. This will allow banks to segment customers and respond to their specific needs and circumstances accordingly and without bias.
Partnering with the experts
Financial institutions taking their first steps on the digital transformation journey would do well to partner with technology and analytics experts, in order to harness the full power of machine learning and AI whilst navigating the various possible road bumps. Experienced partners will also form an objective and holistic view of the current technology infrastructure, which can be difficult to do from within the organisation for a variety of reasons. They can then support the development of a roadmap with clearly articulated deliverables around implementation, monitoring, auditing and optimisation.
This ultimately helps banks and financial institutions to harness machine learning and AI to deliver a range of benefits while protecting them from issues that might inadvertently arise. This also means peace of mind for finance leaders who might be reticent to adopt machine learning for more complex journeys or processes.
The industry is storming towards digitisation whether financial institutions choose to keep up or not. The best advice is simple; find a credible, knowledgeable partner and allow them to light the way to an efficient and responsible data and analytics strategy in 2023.