Steve Morgan, Banking Industry Market Lead at Pegasystems
Artificial intelligence (AI) and machine learning (ML) should both become core components of a banks’ backend operations to enable them better to adapt to changing market and economic conditions.
Simply put, AI uses computers to complete tasks much quicker than humans can, like data analysis, which would take humans hours or even days to do so. One of the aims of AI is to recognise patterns in data sets and recommend the next best steps, making sound decisions almost instantaneously. A subset of AI is ML, which uses data and algorithms to mimic the way humans learn and so enable automated processes to adapt to change, spot anomalies, and recommend changes.
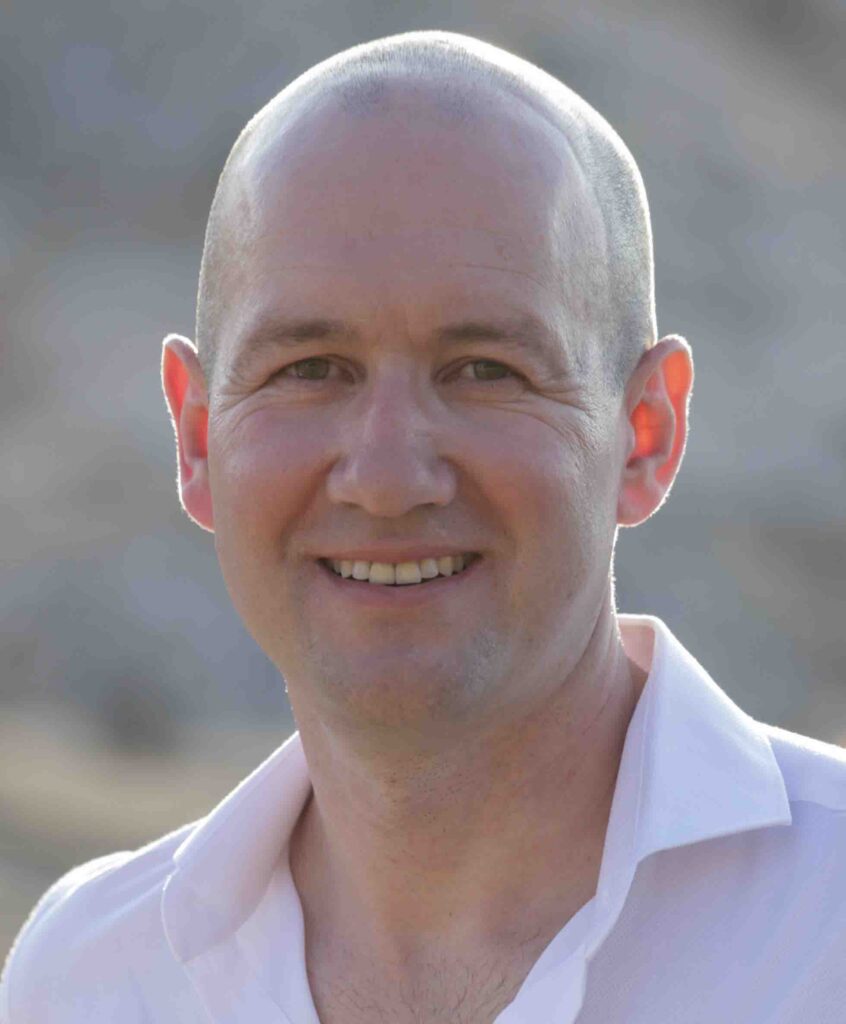
Creating operational resiliency with AI and ML
Most banks have legacy systems in place that can seem to stand in the way of introducing cutting edge technologies. But they should not be put off from deploying the latest versions of AI and ML into their operations as this move does not require a full overhaul of their current backend systems. Through the development of the most appropriate AI-technologies, in collaboration with automation and low code approaches, banks can connect both their legacy systems with these newer technology-first approaches to drive enhanced, up-to-date applications.
Further, as budgets tighten across all industries, banks can enjoy several benefits from deploying AI and ML into their operations. One of the main ones is how AI can automate a growing number of necessary but repetitive tasks, such as data entry. By automating these processes, it can, for example, enable customer service agents to focus on more complex needs which may require a human touch or empathy. This also allows agents to provide more proactive and personalised customer service, therefore improving practices.
Through deploying a best-of-breed AI and ML system, which can be used in conjunction with current legacy systems, banks can significantly reduce their operating costs, and at the same time increase their process and workflow efficiencies.
However, when implementing AI and ML, financial service institutions must be cautious about the ethical and bias issues related to these technologies. To overcome these potential concerns, banks must establish and strictly follow a strong ethical code which promotes non-discriminatory practices for their customers. A way to do this is to train the AI and ML models with diverse data sets and for humans to continually monitor these outputs to ensure that the AI operates as it should. They should also select technology solutions that have transparent and easily explainable models as a foundation.
Using AI and ML for customers
One of the biggest concerns for banks and their customers is becoming a victim of financial crime and fraud. According to a report from Kroll, the majority of businesses expected an increase in financial crime last year; 2024 is unlikely to see financial risks of this kind reduce.
To fightback, AI and ML can facilitate enhanced risk detection and management by connecting case management tools with current fraud screening methods. This approach ensures better protection and security of the financial service institutions’ operations as AI and ML tools are being used to create more efficient detection and management of anomalies, stopping fraud and other financial crime in its tracks.
Moreover, AI and ML not only improves workflow efficiencies, but they can also be used to create financial resiliency for customers. By integrating automation processes into operations, financial service institutions can recommend the best products and solutions to meet their customer needs at the right time. It creates proactive and tailored customer service practices, and they will receive help right when they need it the most. It can also crucially help ensure the right balance of automated compared to in person assistance being delivered at the right time.
The future of financial services is leveraging AI and ML powered automation technologies, which can act as the ‘brain’ of their operations. Using this, banks will be able to streamline their workflows and create operational resiliency and drive a higher and more personalised standard of customer sales and service outcomes.
Whilst many financial institutions have been wary about rushing the adoption of generative AI, implementation of process AI and machine learning continued in 2023 and will grow in 2024. Many banks will move beyond experimentation and into embedding generative AI in specific areas. Other than all the operational benefits that will drive banks to become bigger users of AI, increasing customer acceptance and comfort with AI will continue to help. Are we not all playing with ChatGPT or Bard or another generative AI tool once in a while?